Optical flow for small flying robots
Flying insects heavily rely on optical flow for visual navigation and flight control. Roboticists have endowed small flying robots with optical flow control as well since it requires just a tiny vision sensor. However, when using optical flow, the robots run into problems that insects appear to have overcome.
Fundamental problems of optical flow
Today Nature Machine Intelligence has published our article (featuring it on the cover – [link]), in which we propose a solution to two fundamental problems of optical flow. The first problem is that optical flow only provides mixed information on distances and velocities. This means that using it directly for control leads to oscillations when getting closer to obstacles. The second problem is that optical flow provides very little information on obstacles in the direction of motion. This means that the hardest obstacles to detect are the ones the robot is actually going to collide with!
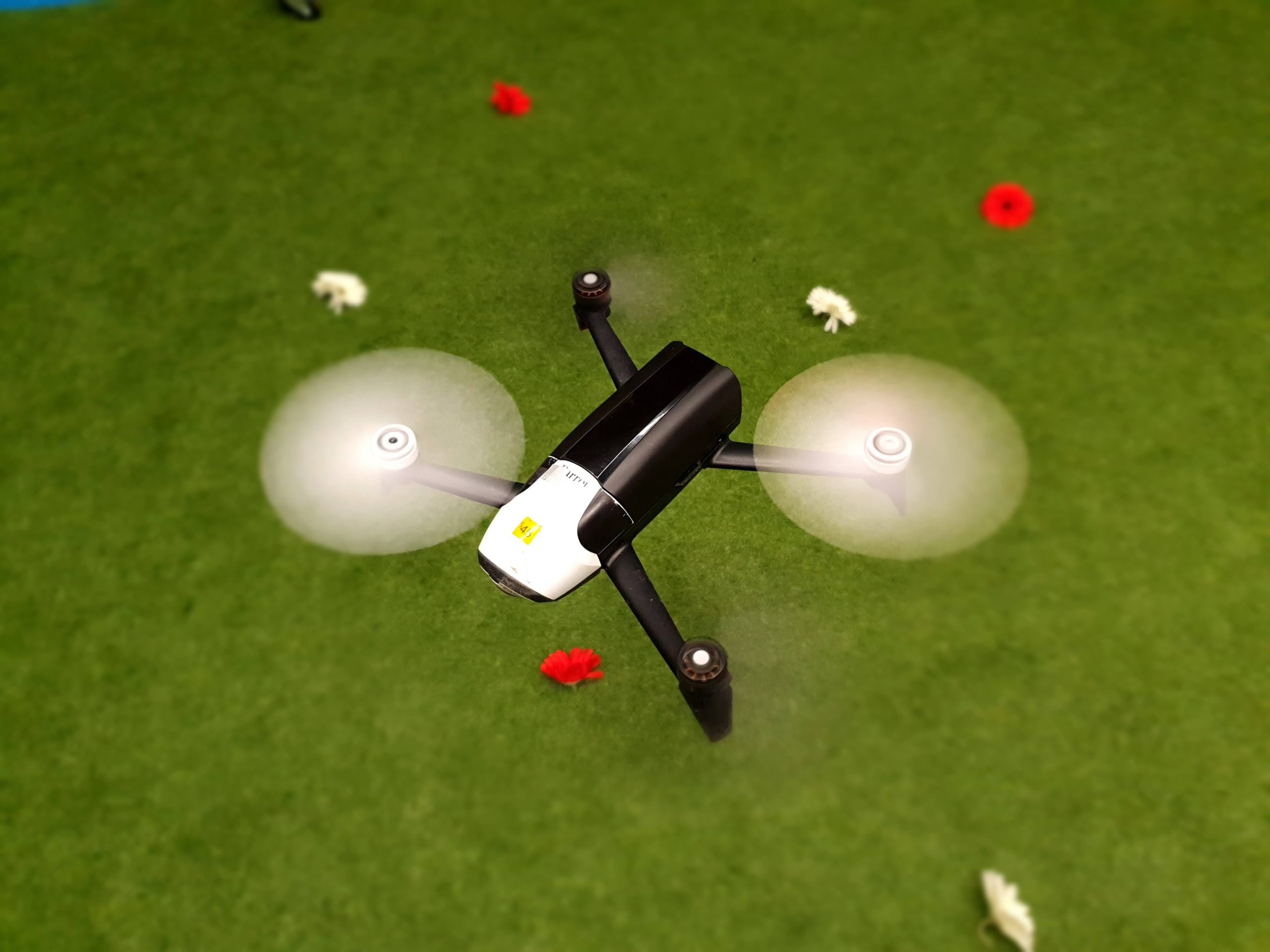
Learning process as a solution
We tackled these problems with the help of a learning process. Specifically, the robot exploits self-induced oscillations to learn what the objects in its environment look like at different distances. In this way, it can for example learn how fine the texture of grass is when looking at it from different heights during landing. For obstacle avoidance, it can learn how thick tree barks are at different distances when navigating in a forest.
After learning, drones can adapt their control to the perceived distance. This results in faster and smoother optical flow landings. It also allows them to detect obstacles in the direction of flight. This results in a faster and safer flight in cluttered environments.
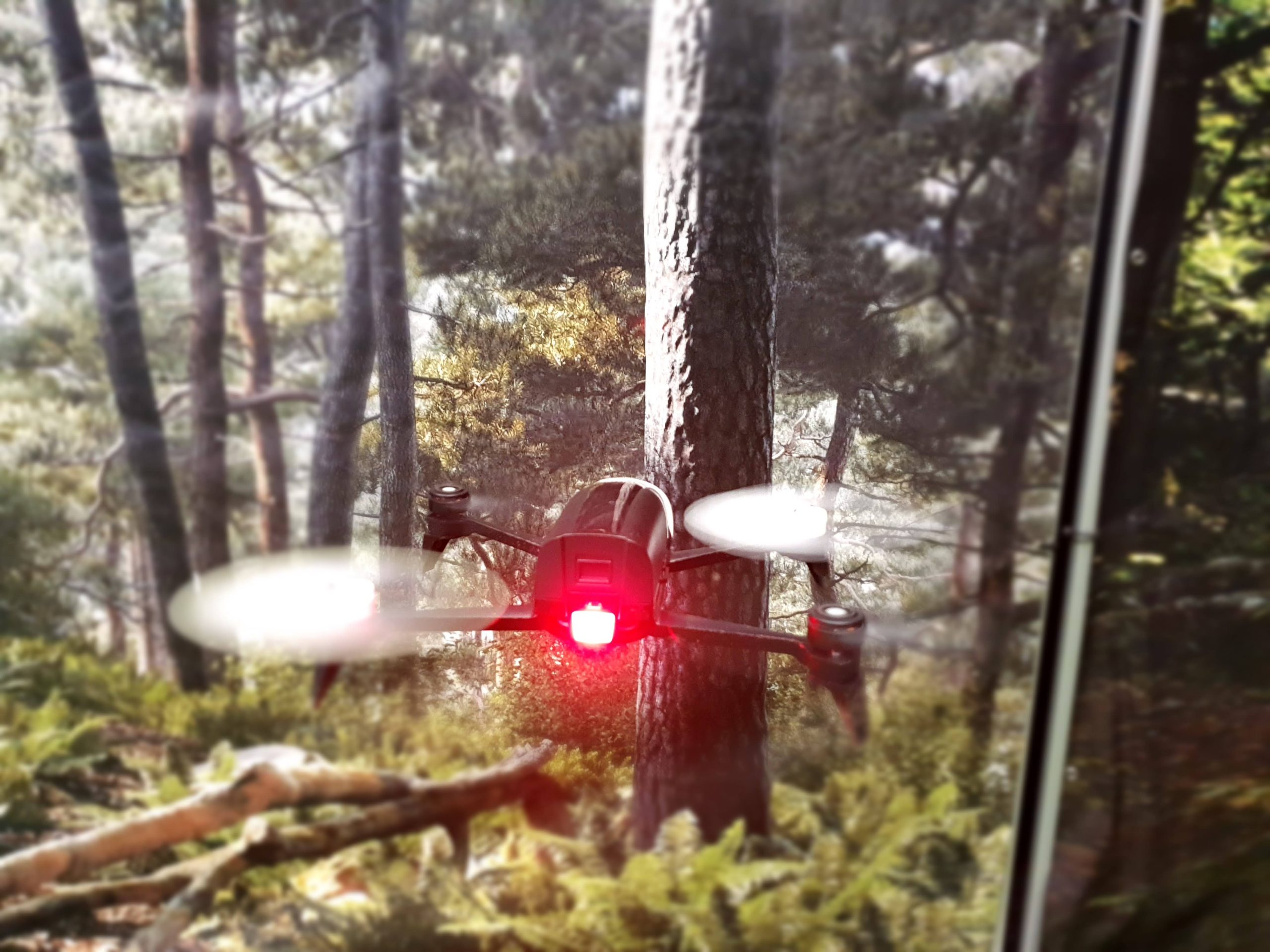
Hypothesis on insect intelligence
The findings are not only relevant to robotics, but also provide a new hypothesis for insect intelligence. Insect flight control for tasks such as landing or obstacle avoidance is typically described in terms of pre-wired optical flow control laws. Our findings suggest that flying insects may learn the visual appearance of their environment to improve their flight and navigation skills over their lifetime.
The article is featured on the cover of Nature Machine Intelligence. Reprinted by permission from Springer Nature, “Enhancing optical-flow-based control by learning visual appearance cues for flying robots”, by G.C.H.E. de Croon, C. De Wagter, and T. Seidl, Nature Machine Intelligence, 3 (1), 2021.
- Press release: https://www.tudelft.nl/en/2021/tu-delft/appreciating-a-flowers-texture-color-and-shape-leads-to-better-drone-landings
- Article: https://www.nature.com/articles/s42256-020-00279-7
- Press media folder: https://surfdrive.surf.nl/files/index.php/s/qxTF9sFW0xEcetd
- Youtube: https://youtu.be/A50Wl311rmU about the solution to fundamental optical-flow problems
Publications
2021
Journal Articles
Enhancing optical-flow-based control by learning visual appearance cues for flying robots Journal Article
In: Nature Machine Intelligence, vol. 3, no. 1, 2021.